This is part 2 of our three-part series on the step-by-step guide to automating the sales funnel with AI. In Part 1, we discussed leveraging AI to automate mundane workflows for the top of the funnel, allowing humans time to pour into creativity and elevate the demand generation game.
In part 2 of the series, we are diving deep into more human elements of the funnel - middle (MOFU) and bottom (BOFU). Traditionally, MODU and BOFU require context-heavy and complex sales and marketing operations. While automation has been part of narrower parts of the funnel for a long time, AI is the new necessary fuel of growth.
Also read Part 3 here.
Mid-funnel Nurturing with AI
Personalized Content Recommendations
AI algorithms look at user preferences and behaviors to suggest relevant content. This often functions like a personalized newsletter directing leads to blog articles, case studies, or whitepapers that are relevant to their interest.
This nurtures leads by offering high-value information. 56% of marketers say targeted content is essential for lead nurturing.
Onboarding Guidance
AI tools provide step-by-step guidance within the app to help users navigate through features. The onboarding experience determines adoption rates and is closely linked to activation, conversion, and retention.
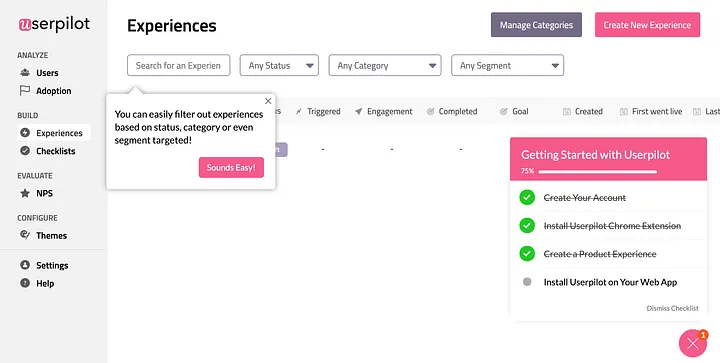
You can also leverage AI to understand product usage and make onboarding guides. You'd still need to edit and validate these guides. For example, this user guide is made by AI.
AI can offer a customized level of training, helping to avoid customer frustration stemming from too long or too short a tutorial.
Lead Scoring and Segmentation
Static lead scoring works - but isn't often correct. It is a lot of guesswork and requires rigorous correlation and multiple revisions to get it right.
Analyzing lead behavior, demographics, and interactions to assign lead scores and segment leads into different categories becomes much easier with AI.
Lead scoring based on ML models does not fit every lead into the same mold but treats everyone as different and provides better conversion signals.
Nurturing Emails and Predictive Sending
If you are still doing date-based marketing nurturing sequences, please stop.
Behavior-based nurturing has proved to be a better way to warm up the leads for closing conversations.
AI helps in organizing the delivery of targeted email sequences. This is an excellent way of delivering the right content at the right stage of the customer journey at the right time. This concept is called Send Time Optimization and it is now widely being adapted by marketing automation software everywhere.
.png)
If your emails are being spam-blocked, AI can also create a workaround by direct mailing personalized emails from a bot instead of a service provider. Here is more info about overcoming bounce rates.
In-app Messaging
AI-powered in-app messaging delivers targeted and personalized messages to users based on their behavior, preferences, or lifecycle stage.
Behavior and usage-based in-app messaging outperform simple, dated sequences. SaaS businesses have seen a 45% jump in activation rates by employing ML-based behavioral messaging.
AI algorithms analyze user interactions and suggest relevant app features or content, increasing engagement and promoting further exploration.
Dynamic Landing Pages
This is a part of Dynamic Content Generation, which includes websites, blog updations, keyword landing pages, ad-specific landing pages, and personalized landing pages for Account Based Marketing (ABM).
Keyword landing pages are linked to specific keyword searches. These help ensure search success and land page relevance per individual.
Ad-specific landing pages create unique pages for each ad and have AI-chosen content selected from your other website content customized by relevance to the specific ad.
You can use Dynamic Landing Pages to affect ABM significantly. These landing pages are created 1:1, meaning the prospect's and their company's personalization tokens will be part of the language on the landing page.
Usage Analytics
ML algorithms can track and analyze behavior, engagement, and conversion paths better than traditional regression-based models.
This data leads to good decision-making a prioritization, as you see summaries of nurturing campaigns, email open rates, click-through rates, and content effectiveness per individual lead.
AI for the Bottom of the Funnel Lead Nurturing
Automated Lead Qualification
ML algorithms' ability to analyze lead data and interactions to determine their readiness for purchase with very little data is remarkable. Hence, businesses have started moving from a one-size-fits-all lead qualification process based on just standard qualification methods.
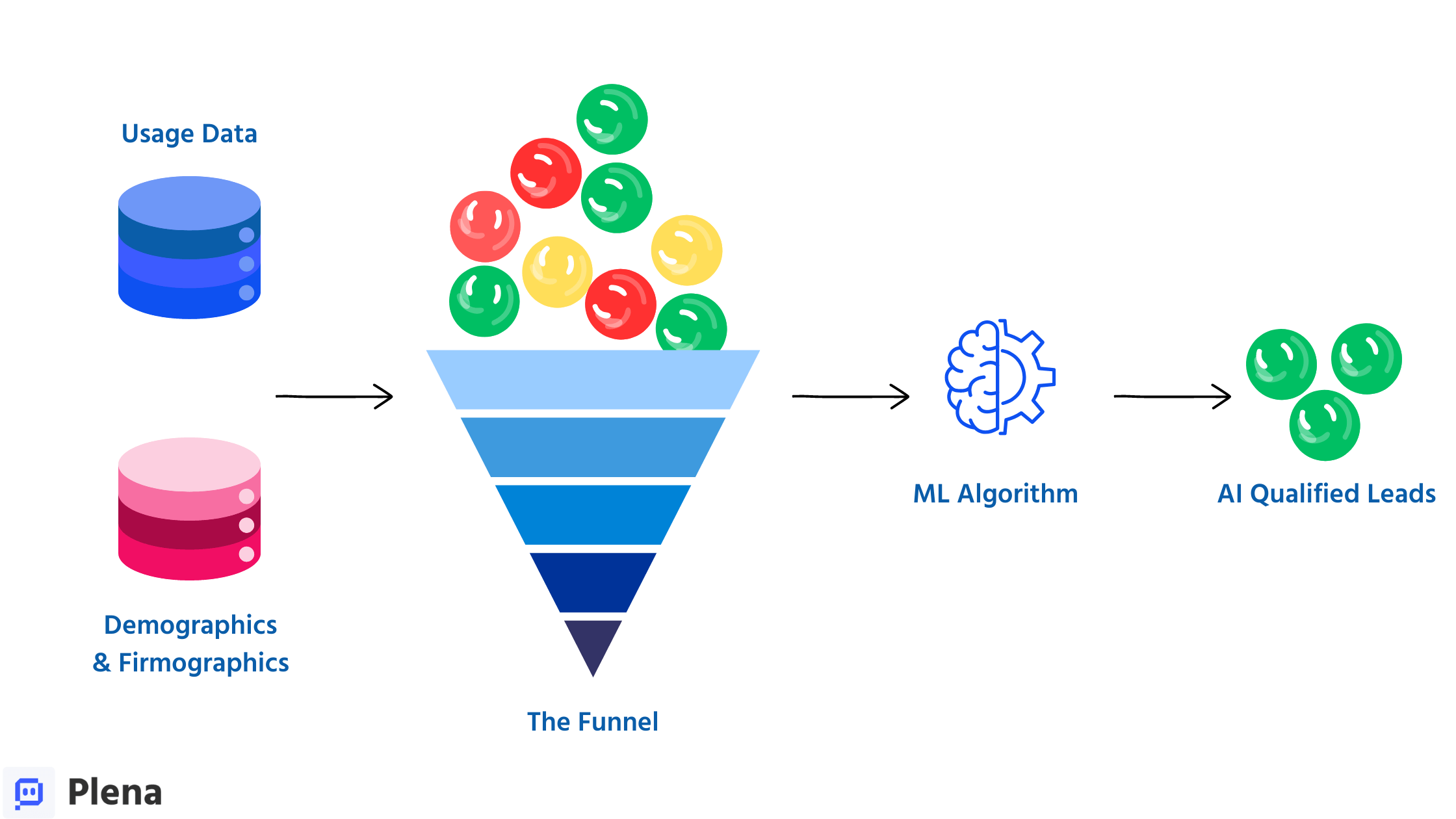
AI helps judge whether they have active intent or are still in the passive intent phase. This helps prioritize high-quality leads, focusing your efforts on the most promising opportunities.
Predictive Lead Scoring
AI algorithms doing lead scoring can predict lead conversion probabilities. This is usually based on historical data, product usage, interaction in communication, and behavior patterns.
Lead scores can also factor in buying intent signals (first-party, second-party, or third-party intent).
Predictive lead scoring help sales teams prioritize leads with the highest chance of conversion and improve their funnel metrics multi-folds.
.png)
Sales Enablement
AI-powered tools provide real-time insights on which aspect, feature, and content your leads engage with the most.
That prepares your sales team for conversation based on real-time insights and engagement.
Based on the history of engagement, AI engines can recommend actions and relevant content or topics to engage with leads effectively.
Personalized Offers and Upgrades
AI analyzes user data to deliver personalized offers and upgrade suggestions within the app. Optimized timing, relevance, and personalization increase conversion rates toward the bottom of the funnel.
Sales Assistants
AI-based sales assistants can provide real-time actionable talking points, summarize call notes, prepare follow-up notes, help in conversion tracks, etc. Like Robin to your Batman.
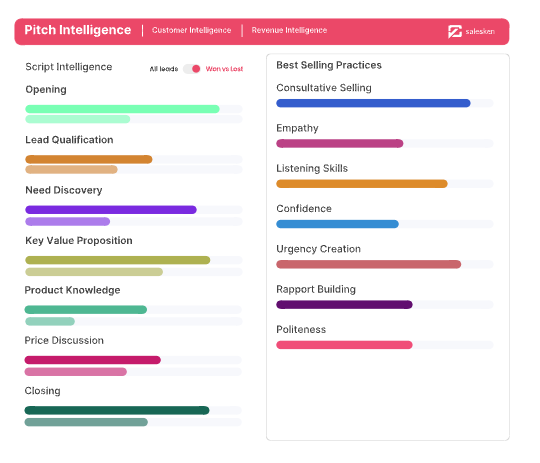
Usage Analytics and Drop-off Predictions
Analyzing usage data, user patterns, and conversion metrics gives businesses insights to optimize the app experience. This identifies user needs enhancing conversions strategies.
AI algorithms also identify patterns and signals indicating potential user or revenue churn. You can launch proactive measures to retain customers and increase engagement.
KPIs and Conversion Tracking
AI tracks lead interactions and conversions and provide valuable data on subjects including but not limited to conversion rates, sales pipeline performance, and revenue attribution. Data-driven decision-making is the best practice.
AI and Customer Success and Support
Conversational AI
AI-powered chatbots handle common customer queries. This usually addresses 80% or more of all questions. Because they provide instant responses and guide customers through troubleshooting processes, customers often come through with a positive experience. This ensures quick and efficient support is available 24/7 in many languages.
AI can understand and interpret customer queries in many languages, 95 in the case of ChatGPT. It results in customer satisfaction and a positive experience as they can use their most comfortable language.
Even if the AI cannot answer the query and passes it to a human customer support agent, translations are available in both directions, allowing an agent to assist across language barriers.
Automated Ticket Responses
Similar to chatbots but integrated into the CxS ticketing software, these AI bots can generate automated responses for common support issues.
Self-Service Options
Related to Chatbots and NLP, AI powers self-service as well. By scraping the knowledge bases or FAQ systems, you can train your models to enable customers to find answers to their questions without human intervention. Don't let your employees spend time on redundant customer queries.

AI for self-service goes across the funnel and plays a crucial role in retention. AI-generated custom walkthroughs for technical troubleshooting are usually superior to support pages alone and save your technical team's energy for systemic issues.
Sentiment Analysis
AI algorithms analyze customer sentiment expressed in support interactions. This helps businesses identify and address customer dissatisfaction appropriately. The accuracy can vary. However, you can use cumulative data in trend spotting and predictive analysis.
After multiple interactions, the cumulative data becomes actionable. Such as all customers reaching out for the same roadblock is a sign of an issue that you may need to update your UX.
Escalation to Human Agents
Knowing when to contact a human is vital to preventing stress responses from customers. AI intelligently escalates complex and sensitive issues to human support agents.
.png)
This transition is often more seamless than when a human agent passes to their manager or another agent because the AI optimally summarizes relevant details for the human agent.
Personalized Customer Success and Predictive Upselling
AI leverages customer data such as purchase history, usage behavior, and patterns to deliver a personalized experience.
You can use these tailored recommendations to identify upselling and cross-selling opportunities, proactively support, and tailor other communications to improve retention.
Intelligent Ticket Routing
AI can categorize and route support tickets to the most appropriate support agents.
The factors used are expertise, workload, and availability, which speed up response rates and decrease ticket close time.
Surveys and Feedback
AI facilitates the collection of user feedback through in-app surveys to identify pain points. Dynamically changing survey flow and questions based on customer behavior, past interactions, and usage drives better response rates and quality of feedback.
AI looks at customer feedback and survey responses to identify areas for improvement. By addressing customer concerns, you retain more customers. The insights are also used to enhance future product offerings.
Customer Support Analytics
Last but not least, ML algorithms analyze support interactions, ticket volumes, response times, and customer feedback. It can also accumulate and report on sentiment analysis trends, escalation rate to human agents, chatbot usage, etc.
Centralized analysis helps you measure and optimize your customer support and success performance. The customer-related part of these data summaries should be reported openly to the company at large.
Allowing other divisions to stay close to the customer experience and journey. This is a core part of encouraging continuous improvement and customer-focused product innovation.
Continued...
This concludes Part 2 of our series Automating the Sales Funnel with AI. In this part, we focused on how the middle and bottom of the funnels leverage AI for lead qualification, activation, adoption, and conversion.
Stay tuned for Part 3, where we will discuss AI's applications beyond the funnels and how it is making waves in the post-conversion, retention, and upselling world.